CogNet AI-MT
AI analysis supported study prioritization for mammography trained on a globally diverse population .
-1.jpg?width=430&height=362&name=Smart%20Worklist%20Image%20Filtered%20(updated)-1.jpg)
Overview
CogNet AI-MT utilizes artificial intelligence to analyze screening mammograms and indicates / alerts the radiologist of cases that suggest the presence of at least one suspicious finding. The suspicious study indication allows for worklist prioritization and clinically determined workflow optimization.
CogNet AI-MT has been developed through collaboration with clinical leaders throughout the world and trained utilizing datasets collected from a diverse patient population in the United States and worldwide (UK, Europe, Middle East, Asia, South America, Africa), supporting the patient and physiological variations that exist in real-world medical imaging.¹
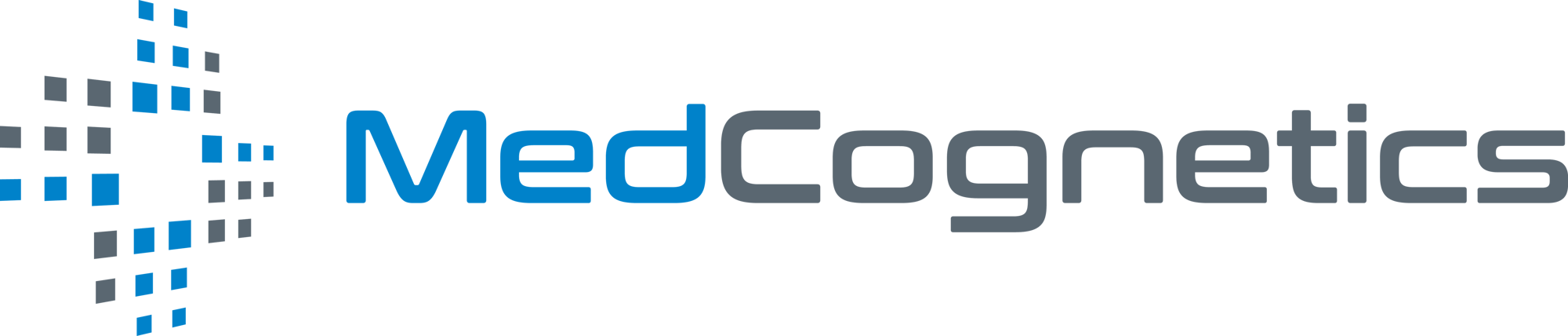
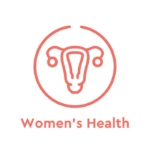
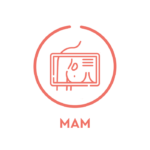
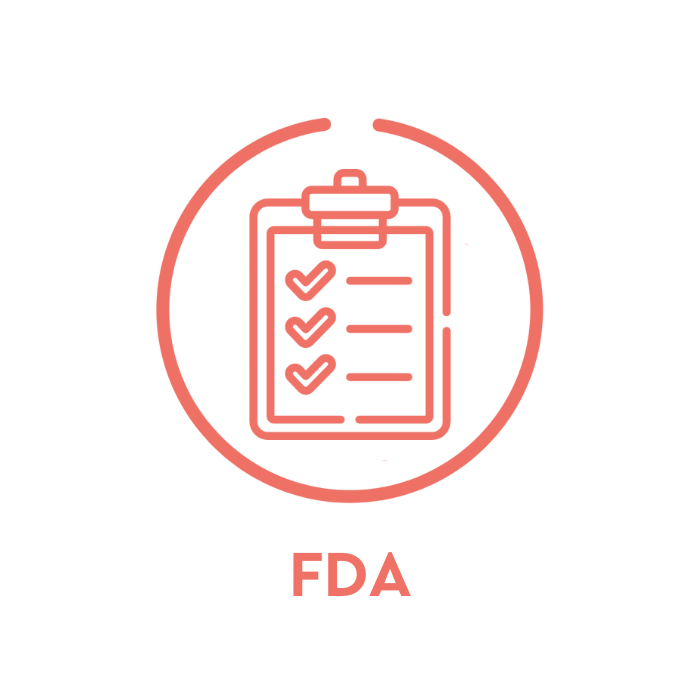
Features
- Suspicious Lesion Indication: The system has an additional filter for catching subtle cancers.
- Study prioritization: analyzes each patient case and provides a case-level classification of 'Suspicious' for the areas that the algorithm identified suspicious areas of interest.
- Filtering: The classification provided to the Radiologist’s worklist allows for smart filtering for prioritization and triage of cases within their list view.
- Integrated Workflow: No extra clicks, keystrokes, or looking at a separate screen. AI results are part of the hanging protocol via DICOM Secondary Capture or DICOM Structured Report.
Benefits
- 'Unbiased AI' – Trained on cases around the world, on a range of ethnicities and races, with the aim that cancer is not overlooked in a patient of any given background.²
- Analyzes mammograms on a pixel-by-pixel basis with the aim to identify small cancers that might be difficult to see otherwise.³
- Having a second validation point to confirm a radiologists assessment aims to increase confidence and peace of mind.
- Having AI results available in their worklist can help radiologists and centers prioritize and route cases accordingly. This may result in the Radiologist determining certain cases being read first, by a senior Radiologist, or even a double read.
- Reducing Operational Costs – Filtering and prioritizing cases may allow for a smart-worklists leading to faster reviews.
To find out more about this solution or any of the other 140+ applications on the Blackford Platform, please book a discovery call with our team.
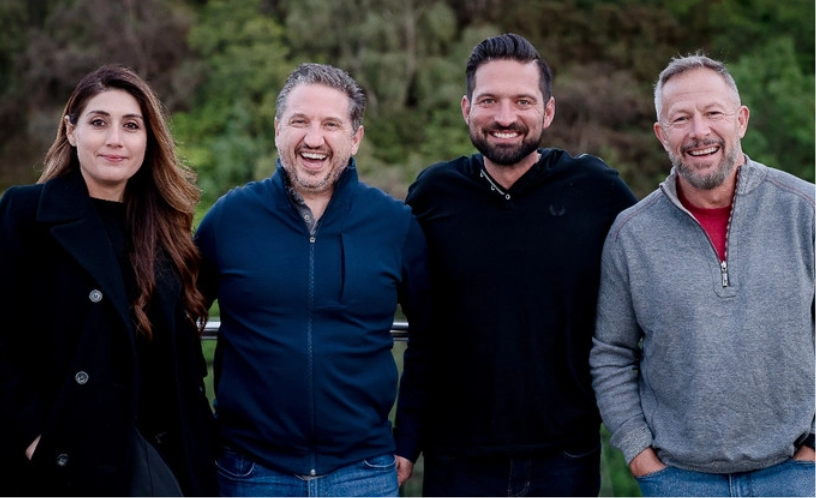
Book a meeting
We’d welcome the opportunity to learn more about your AI needs and to explain how partnering with Blackford can drive efficiency and provide ongoing value.
Book a Meeting¹Abstract: Polat D, Garza SA, Waggener SC, Cogan T, Gupta PS, Garg V, Tamil T, Dogan BE. Bias-Free Artificial Intelligence: Developing a deep learning algorithm for diverse racial populations in breast cancer diagnosis.
²Waggener C, Cogan T, Gupta P, Beaty C, et al. A Racially Unbiased Algorithm for Breast Cancer Detection AIM-AHEAD NIH Project Summary, June 2023.
³Abstract: P. Gupta, T. Cogan, S. C. Waggener, C. Beaty and L.S. Tamil Using an AI Algorithm to Improve the Identification of Small and/or Subtle Cases of Breast Cancer