Lunit INSIGHT CXR
Diagnostic support AI tool that detects 10 common pathologies in a Chest X-ray.
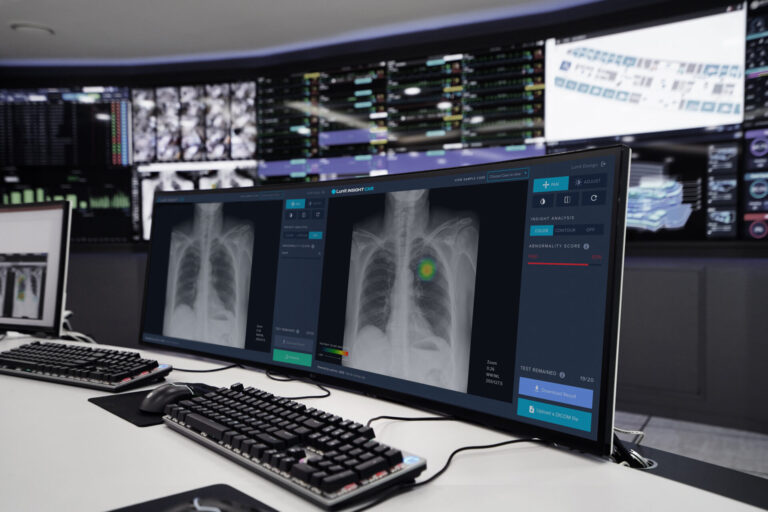
Overview
Lunit INSIGHT CXR is a CADe diagnostic support AI tool that detects 10 common pathologies in a Chest X-ray with high accuracy.

Features
• Findings detected: atelectasis, calcification, cardiomegaly, consolidation, fibrosis, mediastinal widening, lung nodule, pleural effusion, pneumoperitoneum, and pneumothorax. It also supports Tuberculosis screening.
• Compare function available for; pleural effusion, consolidation and pneumothorax.Lunit INSIGHT CXR’s analysis result contains:
• Localization of suspicious areas in color or contour.
• Abnormality Score indicating the probability of the presence of suspicious areas for chest abnormalities.
• Text interpretation for the analysis result per finding.
This solution has regulatory clearance in EU, Canada, Australia, New Zealand, Brazil, Saudi Arabia and more.
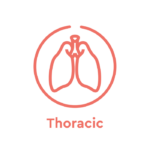
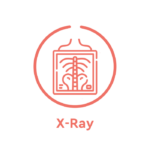
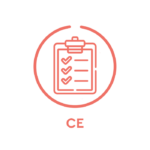
Benefits
• Optimized Workflow: accurately and consistently differentiate normal from major thoracic abnormalities in both acute and non-acute settings (1)
• Improved Reading Performance: better detection of early-stage lung cancer (2)
• Regardless of system, location, or environment, Lunit AI can integrate into the existing workflow.
Image examples
To find out more about this solution or any of the other 140+ applications on the Blackford Platform, please book a discovery call with our team.
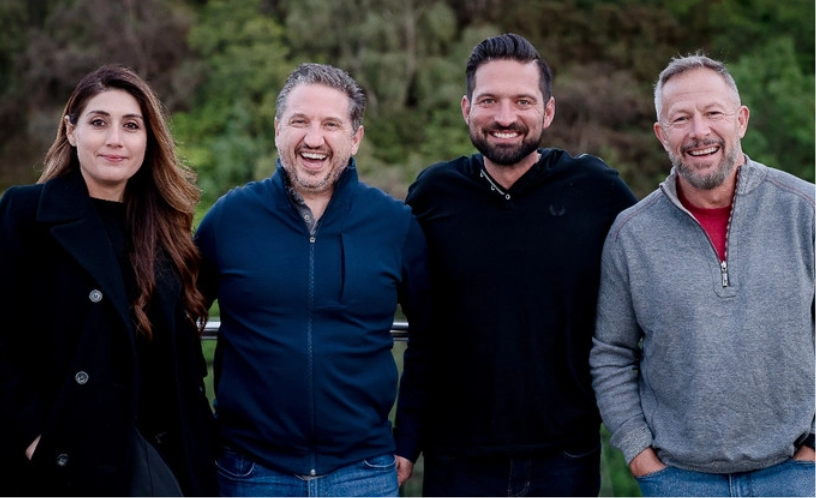
Book a meeting
We’d welcome the opportunity to learn more about your AI needs and to explain how partnering with Blackford can drive efficiency and provide ongoing value.
Book a Meeting(1) Van Beek et al. (2022), Validation study of machine-learning chest radiograph software in primary and emergency medicine – Clinical Radiology, Edinburg Imaging, Queen’s Medical Research Institute, University of Edinburgh, Edinburgh, UK
(2) Ju Gang Na et al. (2020), Development and validation of a deep learning algorithm detecting 10 common abnormalities on chest radiographs