Overview
Deep-learning AI technology, designed to increase CT image clarity, even with ultra-low-dose scans. It uses a deep convolutional neural network, trained to work in a vendor-agnostic way, to reduce noise and enhance image clarity for low-dose and ultra-low-dose DICOM CT images. Trained with over one million patient images containing varying degrees of noise for different body parts, ClariCT.AI’s Clarity Engine separates image noise components while enhancing underlying structures; thus, providing clarity restored images.
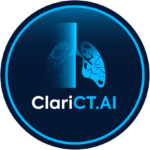
Features
- Fully automated operation without user intervention.
- Fast processing speed, promoting efficient and streamlined workflow.
- DICOM compliant for seamless integration with existing CT equipment and PACS.
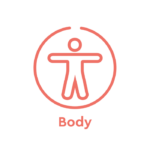
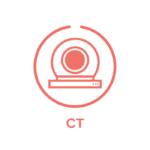
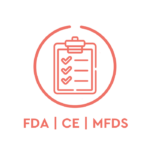
Benefits
- Noise-cleaned images aim to reduce time of CT image reading and diagnosis.
- By lowering CT scan radiation doses, harmful radiation is minimized for operators and patients. Particularly an advantage for radiation sensitive patients, oncology, and paediatric patients as well as those in need of frequent CT scans such as low-dose lung cancer screening.
- Lowering radiation doses also reduces wear and tear on X-ray tube. A study shows that it may extend the life of the X-ray tube by 4 times longer; thus, decreasing costly tube replacement. (1)
- Works with any brand of CT scanner and PACS, old and new.
- Produces high quality CT images with even older/refurbished CT scanners.
Image examples
To find out more about this solution, or the 100+ other applications on the Blackford Platform, book a discovery call with our team.
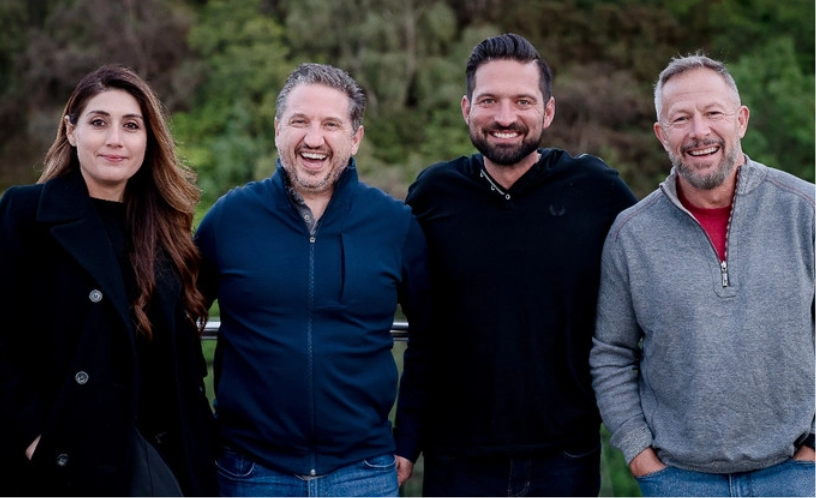
Book a meeting
We’d welcome the opportunity to learn more about your AI needs and to explain how partnering with Blackford can drive efficiency and provide ongoing value.
Book a Meeting(1) Marcovitch A. The Effect of CT Scanning With Reduced Dose on the X-ray Tube Lifetime [Internet]. 2010 [cited 2023 Sep 4].